Experiment like a Data Scientist
Dear Readers,
New tools are making it easier than ever to run product experiments. I'm a lead designer at one such startup called Eppo, which also aims to build an experimentation culture through collaboration. It's a dance between disciplines to run a rigorous and conclusive experiment.
In this newsletter, I will focus on what data scientists wish growth designers knew based on the numerous interviews I've conducted with data teams at high-growth companies. The goal is to help you think more like a data scientist.
Megan Matsumoto, Lead Designer Eppo
#1 - Test one thing at a time.

Test one thing at a time in order to learn and understand which changes cause which effects.
Let’s say you want to optimize your sign-up flow. Users drop off at each of the 5 steps, limiting the number of users joining your product. You look at each step, do some usability testing, and design new variants to avoid roadblocks in the old designs. You could run a test comparing the current experience to your new flow and measure how many more users get all the way to completion. But then you’d lose a great opportunity to learn why your changes helped.
If you tested each new step separately (either running experiments one after another, or in parallel at the same time), you could see how much each individual change helped. Perhaps your changes helped 3 steps yet hurt 2 steps—you still ended up ahead, but if you’d tested each change separately, you could have seen even more gains by only shipping the changes that worked.
One of the changes might have had surprising benefits or problems that can be applied to other parts of the product. Isolating specifically what works and what doesn't is key to scaling the impact of experiments beyond the immediate test.
#2 - Sample size and duration matter.
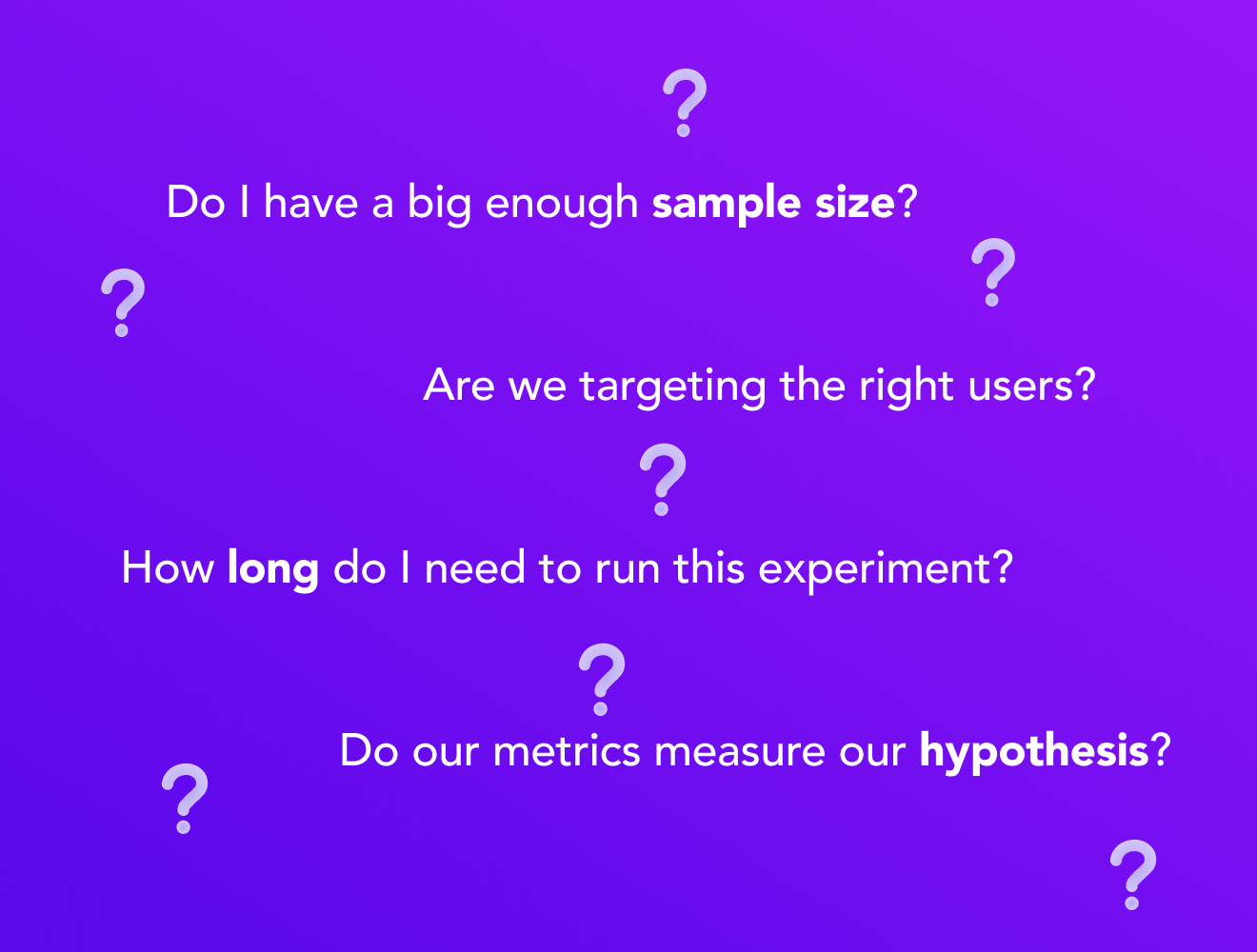
Know when to experiment, and when not to experiment.
You need a large enough sample size and clarity around your metrics for a useful experiment. You may need to run the experiment longer than you think depending on where the change is in your product, as well as the metrics you’re measuring.
My Eppo colleague, and former growth data scientist, Brian Karfunkel puts it well:
“Running an experiment that’s doomed to fail—that is, to not collect enough users to find an effect even if it exists—is a waste of time, but it also erodes confidence in the entire experimentation platform.”
— BRIAN KARFUNKEL, EPPO STATISTICAL ENGINEER
As a team, you should discuss whether it’s worth it to run an experiment for 2 months to influence a single decision.* A Data Scientist can help with that decision process.
*There are statistical methods that can help reduce experiment run time. See CUPED.
#3 - Don’t ship at the first positive signs.

Chart a decision-tree path for shipping, and stick with it.
Yay, you have an experiment that's showing a positive impact on a product metric! When do you ship the change? Right away? After a certain margin? Decide what the criteria is for incorporating a successful experiment into the product before you run an experiment. Doing this up front will make the decision process after the experiment concludes much easier. It also helps defend from the highest-paid person’s opinion (HiPPO) taking over.
Of course, sticking to the plan can be easier said than done. Having sat in a lot of experiment result review meetings, Karfunkel says:
“Once you’ve built the thing, and gone through the energy to test it, and waited for the data to come in, it’s always tempting to just ship it, even if the results aren’t quite what you’d wished for. But shipping something that doesn’t actually work better will hurt your users and your company, and in the end it will hurt you as well.”
I know how frustrating it is to stick to a research plan when there’s so much pressure to ship. The temptation is real to push an experiment as a finished feature at any positive sign of results. In these moments, data scientists are often the voice of “wait and see.” They’re doing this to protect you, not block you.
I invite you to sign up for Eppo and see how the world's fastest-growing companies run faster, better experiments.
About the Author
Megan Matsumoto is Lead Product Designer at Eppo, designing an experimentation platform for the modern data stack. She is passionate about working on tooling that helps teams and organizations make data-informed decisions.
Newsletter edited by Molly Norris Walker.